Modern self-driving cars utilize advanced artificial intelligence technology to recognize road conditions and make decisions. These autonomous driving systems demonstrate excellent performance in dynamic and changing environments through reinforcement learning.
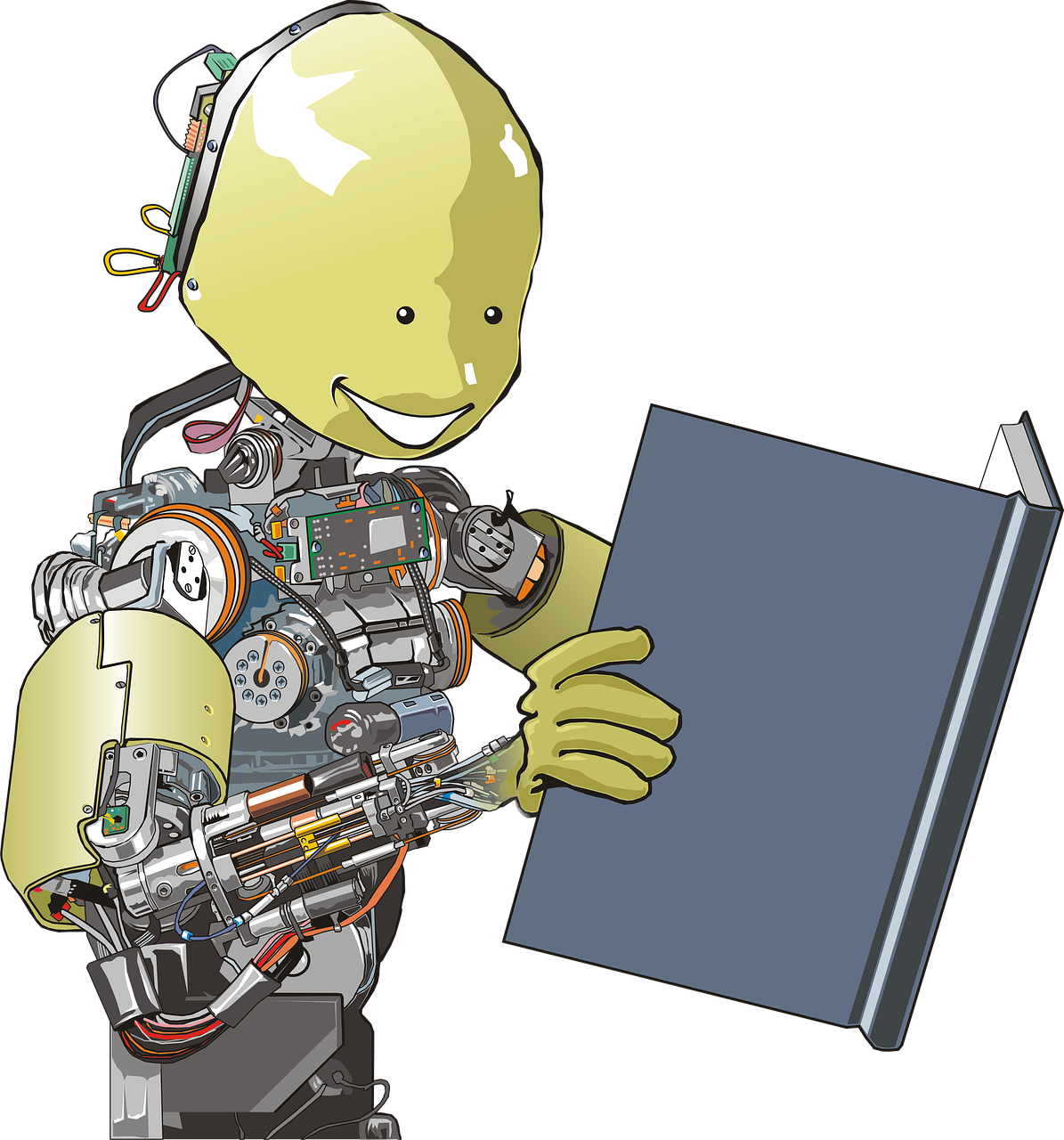
Applications of Reinforcement Learning
1. Environmental detection and state analysis:
Self-driving cars continuously sense their surroundings using various sensors. Radars, cameras, LIDAR and Xyda sensors are used, and the information collected through them is used as input to reinforcement learning models.
2. State space and action decisions:
Reinforcement learning is used to learn the various actions a self-driving car can take and the optimal action in each situation. The state space represents the current state of the car, and selecting actions in each state determines the optimal behavior.
3. Reward system:
Self-driving cars are rewarded for performance, such as achieving defined goals or maintaining safe driving. For example, driving safely, arriving at your destination correctly, and following traffic rules are eligible for rewards.
4. Learning and policy improvement:
Self-driving cars perform experiential learning through reinforcement learning. Initially, learning progresses in the direction of exploring the environment through random actions and maximizing the rewards obtained. Policies are continuously improved to ensure efficient and safe driving.
Field application examples
1. Real-time traffic flow response:
Autonomous driving systems based on reinforcement learning can optimize routes in real time based on traffic conditions. In sections where traffic congestion is expected, you can drive smartly by finding an alternative route or using traffic signals efficiently.
2. Response to sudden weather changes:
When weather conditions change rapidly, self-driving cars using reinforcement learning adjust their driving strategies according to snow, rain, fog, etc. For safe driving, you can reduce your speed or use the driving assistance function using sensors in thick fog.
Reinforcement learning is being applied in a variety of fields, and below are some relevant examples:
3. Self-driving cars:
- Self-driving cars use reinforcement learning to learn from different traffic situations, optimize driving routes, and drive safely. Research is also underway to train models using reinforcement learning environments such as OpenAI's Gym.
4. Robot Control:
- Reinforcement learning teaches robots to perform tasks effectively in their environment. It is used in control of robotic arms, robots that walk on legs, collaborative robots, etc. to improve adaptability to real environments and increase work efficiency.
5. Financial field:
- Reinforcement learning is being applied to complex decision-making problems in the financial field, including stock trading, portfolio management, and risk management. Agents are trained to predict market trends and optimize investment strategies.
6. game:
- Reinforcement learning has been used in a variety of games. AlphaGo is a prime example of applying reinforcement learning to beat the best human players at Go.
Resource Management:
- Reinforcement learning is also used in resource management fields such as energy, water, and transportation to make optimal decisions and utilize resources efficiently.
Medical field:
- Reinforcement learning can be used to improve decision-making and optimize patient care in the medical field, with applications in disease diagnosis, drug discovery, and patient condition monitoring.
These field application cases demonstrate the diversity and flexibility of reinforcement learning, and application in more fields is expected as the technology develops.
conclusion
Reinforcement learning is one of the key technologies that enable self-driving cars to drive safely and achieve their goals efficiently even in diverse and dynamic environments. Through continuous learning and adaptation, self-driving cars are expected to demonstrate a high level of intelligent response to the challenges of their driving environment.
Next- [ChatGPT and AI] - Cloud Computing: The Key to the Digital Revolution
Cloud Computing: The Key to the Digital Revolution
Hello! Advances in computer and Internet technology have significantly changed modern society. At the same time, demand for the way data is stored and processed has increased for both companies and individuals, and one of the keys to this change is 'cloud
sarahspace.tistory.com
'IT 인터넷 > ChatGPT and AI' 카테고리의 다른 글
Big data and cloud computing (0) | 2023.12.14 |
---|---|
Cloud Computing: The Key to the Digital Revolution (0) | 2023.12.13 |
Comparison: ChatGTP and existing search engines (0) | 2023.12.10 |
AI Sensor:Self-Driving Cars (0) | 2023.12.09 |
Introducing ChatGPT: AI Opening a New Dimension in Language (0) | 2023.12.09 |